Data is the new black, or maybe more appropriately the new gold. If you’re in the business of doing business with data, it’s often the last thing you’ll want to give out free. But that might be just what you have to do.
My good friend Hugo Gävert gave a talk in a data science meet-up a while ago. He made a really good point about the need for transparency. Even if you can show a data model has provided undeniable benefits, it needs to be broken down to digestible bits. The Client – your boss, marketing department, another company, whatever the situation is, is sceptical. Maybe even a bit scared of your numbers. You have to convince them, and transparency is the key.
Transparency in the data business, what is it?
The New York Times happened to publish a piece on AI and transparecy just last week. I found it a good read with many anecdotes and interesting bits of history. Long story short: we’re getting to a point where it’s impossible for humans to explain what AI:s do. The solution is of course another AI, which will break it down for us, leading to a recursive process of involving more and more AI:s.
It’s quite futuristic. Here I want to look at something more concrete and down to earth. I briefly touched on this subject in my last post, so let’s expand on those points a bit.
How do you gather data?
In some cases it’s self-evident – when just looking at a web page’s source code you will see what gets sent and where. There are freely available tools that will help you. When working with people data, the General Data Protection Regulation, GDPR, is basically making it criminal in the EU to not be transparent about what data you have.
Although it will definitely cause a lot of headaches for the data business, GDPR is fundamentally a good thing: the right of privacy can be seen as an extension of universally accepted human rights. But only time will tell what the actual outcome of those regulations are.
Anyway, be it people or things, the mechanisms of collecting data are fairly simple. But in many cases there is a big human element: making sure you’re not collecting garbage, missing anything or getting hidden biases. Probably someone will soon give me a counterexample, but machines are bad at taking into account things they don’t see or haven’t been designed to understand.
What exactly do you do with it?
Arriving at a conclusion about data involves a logical sequence of steps. And usually the intermediate results have at least some kind of intuitively understood property. Your client will have an idea of what it means, although they may not understand it completely. Visualising is a very useful tool here, maybe even more useful than visualising the end result!
Another well known and useful method is to reverse an analysis process from result to data. Examples are a powerful way of communicating. Showing the best matching input data for a given conclusion should give an intuitive undestanding that the system works. Otherwise you might want to double-check that your model is doing what it’s supposed to.
How good are your results?
Benefits over not using data are usually quite easy to show. You can compare with acting (hypothetically, although obviously nobody does) at random. Evaluating benefits over a human expert, or another data model or AI for that matter, may be more difficult. But many problems are still quantifiable in this sense. Some sort of success metrics are mandatory for a successful data project.
But it’s worth keeping in mind that even simple numbers are difficult. In the NY Times article, a 60% result for humans is barely better than a coin flip, and an AI excels with as high as 91%. We’re talking about being able to tell a person’s sexual orientation from their online dating profile picture.
I have no idea what those numbers mean! How do I score if I play it safe and assume everyone is heterosexual? I’m sure that the Stanford research group responsible for the results has answers, even multiple ones to a single question. They just can’t go throwing impressive-looking figures around like journalists can, and neither can any data scientist.
How can I influence it?
The client is the best authority on what is usable and needed. Use that to your advantage. Give control to the domain expert to fine-tune the system, model or process. That is the ultimate transparency.
But remember that with power comes responsibility. We want to help the clients, not let them make bad choices on our behalf. That’s where metrics, reverse results and a general understanding of the process can be really useful.
But where is the business then?
I used to think it’s ownership of the data. With people data that’s pretty much consolidated to the big players, even if that’s being challenged with GDPR. In IoT applications, you might own some specialised data gathering instruments. But sensors and Arduino boards aren’t exactly esoteric knowledge these days. Public institutions are more than willing to open up their data for the people. We might as well take for granted that “there’s data”.
Processing power is a commodity. You can get data tools for free, with only a modest effort required to learn to use them. Even the math may be relatively simple, so it’s not 100% sure the old saying “it’s easier to make it work in practice than in theory” applies either.
The real question is, does it make any difference if you have the data or not? Can you use it? Actionability is the core of the data business.
Without thinking, is hard!
That’s what I wrote in my notebook during Hugo’s talk. I can’t remember what his context was exactly, but thanks buddy, well said! I know I’m always trying to make things sound easier than they are. But there really is a hard part with data: actually making it easy to use it.
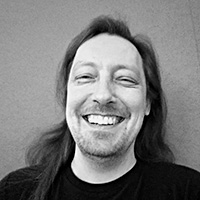
Pirkka Kärenlampi
CEO
Pirkka is a senior software consultant and the CEO of Creacomp.
He likes asking questions more than stating facts, simplicity more than complexity, quickly testable hypotheses more than carefully laid out plans. He’s here to make things happen.